When a financial blogger puts a word like “ontic” in the title, they not only sound pretentious, but perhaps the best way to ensure nobody opens those emails. Yet, here we are. After the first part of this series last week on the philosophical, epistemological aspect of the new Math, this one almost begs to be written. We promise two things:
- We will keep the focus on investment-related conclusions on this note; and
- We will return to the usual real event-driven topics in the next note and never use words like “epistemic” and “ontic” for a long while, and maybe forever.
After all, our world has new inhabitants. They don’t breathe, but they listen. They don’t see, but they parse. They are not alive, but they act. We cannot pen a poetic ode to their silent hum, but the age of intelligence deserves one more article on the practical parts, particularly in these quiet months when clarity feels more elusive than ever.
In summary, our previous exploration (link to original article) was about the epistemic shift, in the philosophical parlance, how these models transform our ways of knowing, from taxonomies to fluid abstractions. But there’s a deeper and practical layer to this revolution that beckons us to ask not just how we know, but also what is. The Structured-to-Unstructured (S2U) shift, powered by transformers, is not merely changing our understanding but redefining the very nature of reality as we have known it. We are in the world of machines where patterns, relationships, and entities emerge not from human design but from the machines’ multitudinous gaze upon the digital deluge. Volumes will be written on social, moral, ethical, economic, and other aspects of the emerging world, while in this note, we will try and keep returning to what this means for investors in the way they think about innovation and growth investments.
The Ontic Shift: Reality Through Machines’ Eyes
Last week, we explored how transformer-based models reshape work processes by transitioning from structured to unstructured data handling, fundamentally altering how we interact with information. The best way to understand what is changing with “what is” or how reality is rewritten is by looking at the well-known plight of traditional search.
Apple’s confirmation last week made it official that Google searches are down for the first time in over two decades. Reports on website traffic stats imply a 20-50% fall for most sites due to AI replacing the need to visit websites for basic answers. Information no longer flows straight from the horse’s mouth—not even in the figurative sense of yore—but through the inscrutable filters of AI models, processing and distilling reality before it reaches us.
This filtering happens across all that we consume. At GenInnov, call transcripts, press releases, and annual statements are no longer pristine documents we pore over with coffee. They’re processed by LLMs first, distilled into sentiment, risk, or growth patterns. Even conference calls are not spared: the GenAI analysis of nuances and differences is so rich that one rarely comes across a note from an analyst these days that offers anything significantly new. This is the ontic reality on the receiving end: what exists for us is increasingly what the machines present. And, as we’ve noted before, the creation side is already in AI’s hands—models drafting those very press releases or earnings summaries.
Machines in the Loop: The New Architects of Reality
We most certainly do not mean humans are entirely out of the loop. But what’s changed is our role—and more importantly, the increasing authority of machines in defining what counts as an event, an insight, a movement. This is not a story of full automation; it’s about epistemic crowding. Machines are increasingly upstream in the decision process. It is futile to argue how much role machines are playing in shaping the reality as of today. What is given is that as machines share more cognitive elbow room, their influence is going to increase.
Transformers reveal a different type of existence, which means a company’s market perception at the end of an announcement now hinges more on how these models are likely to interpret it than on how humans alone perceive it. For instance, a company’s market perception at the end of an earnings call is less about what was said and more about how transformer models parse tone, nuance, and forward guidance.
For corporate IR teams, this is a seismic shift. It’s no longer just about crafting a press release that resonates with analysts or shareholders;It’s no longer just about crafting a press release that resonates with analysts or shareholders; it’s about ensuring that transformers interpret it in ways that align with the intended message.
This isn't just IR. It's everywhere:
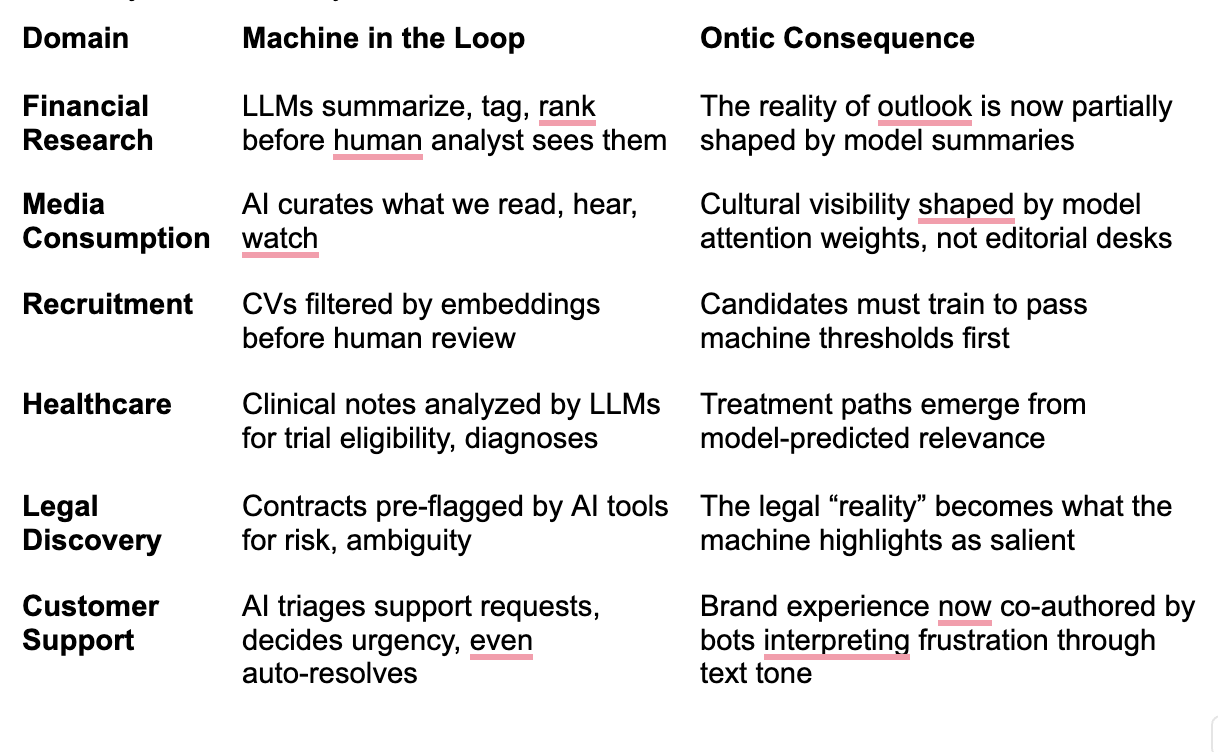
The Illusion of One AI: Combinatorics, Chaos, and the Investment Conundrum
If machines are now reading earnings calls, summarizing the news, decoding tone, and pre-flagging corporate risk, why should not we just let them run the portfolio, too?
The answer is simple: there is no such thing as one AI. And therein lies the mess. The issue isn’t about trusting AI to decide but choosing which AI to trust. And that’s a harder problem than most investors realise.
This brings us to the concept of a combinatorial explosion. Consider the building blocks of life: just a handful of atoms, like carbon, hydrogen, oxygen, and nitrogen, can form around 10^60 different molecular combinations. From this vast possibility, only a tiny fraction gives us RNA-based life or, say, a cancer cure.
This combinatorial chaos isn’t limited to science—it’s a pattern we’ve seen in finance before. It should have wreaked havoc on passive investing, too. With the arrival of computers, it became possible from a global pool of a few tens of thousands of listed stocks to create a vastly higher number of indices, baskets, ETFs, and the like. In fact, we also saw a proliferation, which should have made choosing the right ETF far more difficult than picking individual stocks. The decision space had ballooned for the passives. It did not matter in hindsight; as the biggest markets and their largest stocks and sectors outperformed consistently for two decades, the best pick turned out to be the simplest combinations created in the pre-tech era.
Likewise, a vast decision landscape unfolds from a relatively small set of input facts in call transcripts, price trends, sector comps, and geopolitical sentiment. Each model interprets differently. Each prompt nudges differently. Each pre-training corpus carries its own biases. There is no one LLM. There are infinite embeddings, fine-tunings, architectures, modalities, and reward functions.
And so, unlike legacy quant investing, where one might argue about the relative opacity of a factor model, here you’re dealing with a fractal explosion of decision matrices that change based on how you phrase the question.

The point is that in traditional quantitative investing, the model maker could retrace and determine how a decision is made. In fashionable “AI-investing”, and here one has to expect an explosion of products, it will be black box, faith-based investing to the next level, where even AIs themselves cannot reproduce the factors or the outcomes. In the old world, too many ETFs were a financial innovation feature. In the new one, too many LLMs are a bug of interpretive chaos. The ontic reality isn’t that AI tells us what is: it’s that each AI sees a different "is." The worse, the same AI sees a different “is” at different times.
Living with Infinite Beings
A haunting quip floats around tech circles: AI might just be the last innovation humans ever make, a final bow before machines take center stage in the theater of existence. From here on, they’re not just tools but the heart of what “is,” shaping realities we once thought were ours alone to define. Our language scrambles to keep pace, dressing their actions in human terms. We call them “agents” now, as if they might join us for a coffee break, long before humanoids populate our physical world. In this Structured-to-Unstructured data shift, which we’ve termed S2U, every domain of activity faces upheaval for years to come.
In the coming years, scholars, lawmakers, and communities will produce insightful books, legal frameworks, social guidelines, and handbooks addressing the ethical, psychological, and spiritual challenges of coexisting with machines that surpass our cognitive abilities. But this note has a narrower purpose: what do participants in financial markets do differently now?
History offers some clues. When calculators arrived in the 1970s, log tables vanished, but accountants simply evolved as they stopped recalculating interest tables and started focusing on judgment and forecasting. When spreadsheets became ubiquitous, analysts stopped drawing by hand and started modeling scenarios. Now is a similar moment, with a twist. The human job is increasingly less about producing the base material. Though the habits of attending calls and creating decks won’t vanish overnight, the real value lies in something else: guiding, interrogating, contextualizing models, and above all, not being tricked by them.
Using the mundane example of what we at GenInnov do after any earnings call these days:
- We are happy to collect transcripts and any written material, to start with
- Our focus is on asking the right questions in a prompt-savvy way. In nine out of ten cases, we generate output far superior to anything we see coming from the usual channels, even days and hours later
- We try to spot when an AI-generated answer looks too fluent to be true
- We attempt to master when to use GenAI versus when to read the original source.
- And, crucially, the most important thing for us is to verify and critique every important point through counterarguing AIs
At GenInnov, we don’t just plug in models to automate workflows. We prompt, cross-prompt, triangulate, and in many cases, we deliberately use divergent models to see which interpretation holds up across systems. The machines are not just time savers. They are our conviction testers.
In other words, the AI era requires investment investigators to be cognitive choreographers rather than soloists. One who sees models not as unified oracles but as chaotic chorus or at best, debate participants. Smartest users would recognise that even the best insight needs grounding in values, context, and goals. In our case, as innovation investors, this means growth strategies hinge on steering this multitude to uncover opportunities, like a biotech firm’s potential through a treatment financial world analysts may know little about, or implications of a new LLM method for the compute demand.
The Ontic Reality: Living Among Cognitive Clouds
There is more. We now live among cognitive entities that don’t have a body, don’t forget, don’t tire, and don’t sleep. Our models can ceaselessly work in the background on things that do not require human decision-making or any other forms of intervention. We need to learn to change workflows to harness these new capabilities wisely.
We repeat: machines as filters, lenses, agents, and beings, carry profound implications for humanity. Each of us should expect to be repeatedly torn asunder in the coming years by their weight.
This note, of course, was a narrow take. We aimed only to map the ontic contours for those in financial markets navigating this shift. In that sense, the conclusions are simple: design with machines, validate through machines, cross-examine through machines but there is nothing like surrendering judgment to machines, as “AI” is not a singular entity.
The question is no longer whether AI will define the future. It’s whether we’ll stay engaged as it does so in interpreting, questioning, and shaping the path, even as the fog of cognition thickens around us. The answer, like most good investments, depends on staying curious, cultivating judgment, and knowing which fragment of the multitude to trust and when to override them. Used appropriately, this multiplicity can unearth opportunities no single human or team could. A new abstraction. And perhaps, the final lesson from the age of choice: it’s not the machine that sees the truth — it’s the one who chooses to wake up and take the red pill, again and again.